I hope you enjoy reading this blog post. If you want our handwritten notes to drive more sales for you, click here.
The Science Behind Audience.co’s Likely Seller Recommendations
We’re here to explain the science and reasoning behind Audience.co’s ‘Likely Seller’ recommendations, so you understand how we use it to better target homeowners with our handwritten notes and digital-marketing follow up.
In a nutshell, our Likely Seller recommendations help our clients engage with homeowners in their farm and sphere who are most likely to turn into new listings.
Our Likely Seller model factors in data points about each property, neighborhood data, past market trends. We also incorporate data from thousands of QR code scans as a result of sending millions of notes to homeowners across the country.
Examples of factors we incorporate into our Likely Seller model:
- Size of the house, number of bedrooms, lot size and other features of the house and property.
- Home value appreciation compared to other properties in the neighborhood.
- Owner age. For example, owners over 50 years old may well have a higher likelihood of selling
- Number of times the property has sold and years since the last sale. For example, if the last transaction was a distressed sale, it would make the likelihood high of a subsequent sale.
- % equity the owner holds in the property and the status of the mortgage.
- Census tract data for that area (for example, birth rate probability, which could predict a likely seller in a starter home)
Why ‘Likely Seller’ Suggestions in Real Estate?
You must stay top-of-mind with leads and current and past clients to stand out from other real estate agents. Take these statistics into consideration: NAR (National Association of Realtors) reports that “68% of sellers who used a real estate agent found their agents through a referral by friends or family, and 53% used the agent they previously worked with to buy or sell a home.”
However, even though 90% of sellers claim they’d use the same agent again, most of the time, they can’t remember the agent’s name, says Inman.
Maintaining a nurture-based relationship with your farm and sphere greatly improves the likelihood of future business. Yet, without a likely seller model, it’s impossible to narrow hundreds or thousands of homeowners into a list of likely sellers showing where to focus the agent’s time and energy.
The goal of ‘Likely Seller’ recommendations is to provide our real estate agents the opportunity to engage with ideal homeowners primed to sell efficiently. Specifically, the technology predicts properties most likely to sell within 12 months.
We could have developed a thousand different AI projects, so why did we focus on ‘Likely Seller’ recommendations?
- Likely sellers are more likely to be motivated to sell their home within 12 months, allowing agents to nurture the prospect so they won’t forget the agent’s name; they stay top of mind. (In some cases, they may even be motivated to sell right now.)
- Data needed to train the algorithm and assess its performance is readily available.
- Homeowners at the top of the “Likely Seller’ list may refer friends, family, or colleagues who are likely sellers.
- The AI provides a platform for other technologies, including marketing, prospecting, farming, and more, can be built.
- Audience’s tool will likely be even more accurate using unique QR-code-scan data sets from sending a million handwritten notes to homeowners.
These are just a few reasons we chose to explore an AI system that gives our agents a fantastic competitive advantage. We’re constantly working to provide real estate agents with the best tools to succeed.
Digging Deeper Into AI-Powered ‘Likely Seller’ Recommendations
From here on out, things get a bit more technical, so let’s cover a few definitions to help you better understand the technology behind Audience’s ‘Likely Seller’ recommendations. The three main parts of the ‘Likely Seller’ engine are:
- The Machine Learning System: An AI-based algorithm that uses data to create the model.
- The Model: A second AI-based algorithm that suggests likely sellers.
- The Decision-Making System: A system that takes available data and predicts which contacts real estate agents should engage.
So, the machine learning system creates the model, which provides data the decision-making system uses to recommend likely sellers in an agent’s contact list.
The model uses statistical estimation based on household data, a combination of home and homeowner records, to predict likely sellers in the coming 12 months. What home data is used by the AI-driven system?
- Value Appreciation: targeted home value vs. area comps.
- Property Details: property specifics such as total square footage, bedrooms, bathrooms, bonus rooms, and more.
- Sales Data: frequency of and time since the last sale.
- Mortgage/Equity Position: mortgage situation and equity estimation.
- Movement Statistics: percentage of homeowners vs. renters and typical moving patterns.
We then combine this methodology and factors with data from sending millions of handwritten notes to homeowners across the country. We use QR-code-scan data from our sends to identify the factors that increase a household’s likelihood of selling.
The ‘Likely Seller’ system incorporates and processes data in intricate ways. Let’s look at an example that highlights important details about the process.
A property is located in a small neighborhood of 1- and 2-bedroom homes. On average, homeowners live in their homes for four to six years before selling.
Based on a human thought process, the data leads you to believe this is a community of first-time homebuyers who choose to upgrade as their family grows.
The AI-driven system can’t think like humans; they focus on data and probability. ‘Likely Seller’ recommendations need human knowledge and inference to move from recommendation to contact. With that said, data-based predictions may pinpoint likely sellers without additional information.
‘Likely Seller’ recommendations are based on the likelihood a home will sell within 12 months. One house may score 15% and another 5%, but these probability percentages are relative. Depending on sales statistics, a 15% likelihood in one area may be low, while a 5% likelihood in another area may be high.
Here’s an example to illustrate how ‘Likely Seller’ probability works.
Agent A works in an area with a 3% yearly sales rate. A likely sale is recommended with a probability score of 5%. Agent B works in an area with a 15% yearly sales rate. A likely sale is recommended with a probability score of 10%.
Agent A immediately contacts the homeowner to start the nurturing process. A 5% likelihood of selling is above average, thus the excitement to get things moving. Agent B likes the 10% probability, but with a 15% yearly sales rate, they may need to spend more time refining the suggestion before starting the nurturing process because 10% is below average.
Another critical factor to remember is that not all homeowners will be ready to sell immediately. Remember, ‘Likely Seller’ recommendations are based on a 12-month window. Maybe one contact won’t be prepared to sell for nine months, and another may stretch that to 18 months or more.
You also want to remember a homeowner with a 10% likelihood of selling won’t list within 12 months 90% of the time. Contact, however, isn’t futile. The homeowner may very well know several people looking to list within the next few months. Remember, Audience’s ‘Likely Seller’ recommendations may require nurturing with multiple touches over a year or more.
When Does a Prediction Become a Recommendation?
Prediction is the second step in the recommendation process, so how are recommendations singled out from predictions?
The ‘Likely Seller’ system pulls home and homeowner data from a real estate agent’s contact list. This data is fed into the model (as described above), and a score is assigned to the contact.
How high does a contact need to score to be recommended?
We advise our agents that scores in the top 10% hold an elevated opportunity of being a ‘Likely Seller’. However, we suggest focusing on the top 25%, which includes contacts with an average likelihood of a sale.
What does sales data have to do with recommendations?
The percentage of homes sold in a given area for a specific year is called the baseline, and the prediction score must be significantly higher than this to be recommended.
For instance, if an area has an annual home sales rate of 10% and a contact within that area scores 10%, there’s no boost in sales probability.
However, if the area has a 2% annual rate of home sales, the 10% likelihood is super exciting. What once was a 1 out of 50 chance of finding a ‘Likely Seller’ decreased to a 1 in 10 chance. That’s quite the improvement.
Are the top 10% of homes substantially more likely to sell than the bottom 90%?
From our experience at Audience.co, the top 10% tend to sell twice as often as contacts 11% and lower. It’s crucial to note that a 2x boost is the minimum our agents have experienced.
Side Note: Audience’s ‘Likely Seller’ engine is constantly updated and improved with new data points, fresh information, and algorithm updates. New models are regularly tested so our agents can make the most informed decisions possible.
Looking Closer at the AI Behind ‘Likely Seller’ Recommendations
We want to dig a little deeper into the AI-driven aspect of our ‘Likely Seller’ tool. Don’t worry; we won’t throw a bunch of math and statistics at you, but we’ll give you an inside look at how everything happens.
AI-Powered Machine Learning: Training AI to Solve Problems
There’s a lot of machine learning jargon used to describe teaching, training, and testing Audience’s ‘Likely Seller’ recommendations. Instead of smacking you in the face with a bunch of new vocabulary words, let’s address commonly-asked questions.
What is the target variable?
The target variable in AI-driven machine learning real estate prediction models is the likelihood a home will (will not) sell in a future timeframe. Data from past sales are used to train the system. Data from the past five years, for instance, are combined to estimate the probability of a sale in the coming 12 months.
Why is 12 months the ideal prediction timeframe?
Real estate is a volatile market with a substantial seasonality factor. Estimations are made based on the ebb and flow of home sales throughout the year. So, if the model predicts a high likelihood of a sale, it means a sale could occur within the next 12 months, all things considered. One homeowner may wait five months to sell, another nine months, and a third eleven months.
What drivers help predict what homes will sell soon?
Establishing the target variable is the first step in an iterative process. The next step is feature engineering, where the AI algorithm pulls data points on a property to influence predictions. Some data points include:
- Value Appreciation: targeted home value vs. area comps.
- Property Details: property specifics such as total square footage, bedrooms, bathrooms, bonus rooms, and more.
- Sales Data: frequency of and time since the last sale.
- Mortgage/Equity Position: mortgage situation and equity estimation.
- Movement Statistics: percentage of homeowners vs. renters and typical moving patterns.
New correlating data points are continually tested and added to Audience’s ‘Likely Seller’ recommendation system.
It’s important to review the difference between correlation and causation. Our machine learning system doesn’t utilize personal information to predict a sale – it doesn’t estimate based on a cause. For instance, the AI won’t know if there are kids in the home or new empty nesters (causation), but it will know how many years a homeowner lives in a home (in a given area) before selling (correlation).
Wasn’t feature engineering snuffed out by deep learning?
Deep learning has its place in advanced technology, but feature engineering isn’t dead. Our predictive model doesn’t have an influx of training data, which renders deep learning unnecessary. Instead, we focus on data sources to populate information on home sales, size, location, time living in the home, and others. Audience also uses proprietary in-house data based on results from sending millions of handwritten notes. In particular, we incorporate QR-code-scan data from our sends to zero in on the factors that make a household most likely to sell.
Does Audience’s system use cross-validation for holdout evaluation?
Cross-validation doesn’t work with our AI model because it takes a year to collect prediction outcomes. But, from a testing perspective, calculate features at the beginning of training and testing. From one year to the next, models learn from the past year’s sales and use that data for future predictions.
More about training, testing, and predicting:
When we dig deeper into testing and training, we can hone in on how our AI model works. Let’s check out an example from the year 2025.
- Training: Training for 2025 predictions start in 2023. Sales data, among other factors, are used to train the AI. Preliminary forecasts for 2024 are made based on this data.
- Testing: The test period is the year 2024. Based on the predictions from 2023’s data, how well did the algorithm perform?
- Predicting: Each 2025 prediction is based on the results of 2023’s training and 2024’s testing.
What about geographic differences; do they matter?
Geographic differences will undoubtedly impact how predictions pan out. To solve this problem, we’ve broken the US into various regions so that models can learn the intricacies needed for successful predictions.
Remember, “changing the real estate technologies could change system dynamics and improve real estate market transparency. Moreover, it can be asserted that, in a broader sense, proptech is beneficial for territorial competition and territorial growth strategies,” says IOP Science.
Remember, each geographic region is assigned a model, and no single model version will perform well across all regions.
Does uncertain science limit the usefulness of ‘Likely Seller’ recommendations?
Sure, the uncertainty of science plays a role in our AI’s predictive capability. There are no guarantees when it comes to machine-learning projects like this. It remains uncertain if the prediction will come to fruition, and we can’t say with 100% certainty that it will.
One thing we’ve found during testing and training is non-linear modeling outperformed linear modeling in real estate sales predictions.
Can recommendations based on past home data continue to perform over time?
Yes, without a doubt, training core models on previous year(s) data will continue to perform over time if no significant changes or events could throw the entire model off.
For example, a blizzard hit New York City in January 2022, which changed the real estate outlook for that region. ‘Likely Seller’ predictions for homes in the impacted area may no longer be viable. However, the model remains stable when life-changing events are removed from the scenario.
Implementing machine learning for real estate applications is a complex process. We want you to understand the science behind our predictions, so stay tuned for more on Audience’s ‘Likely Seller’ recommendation system.
This article wouldn’t be possible without the incredible work of Foster Provost, Panos Ipeirotis, Eda Kaplan, Nate Rentmeester, and Varun D N, among others. Read more about their work: Likely to Sell Recommendations for Real Estate and Machine Learning in Action.
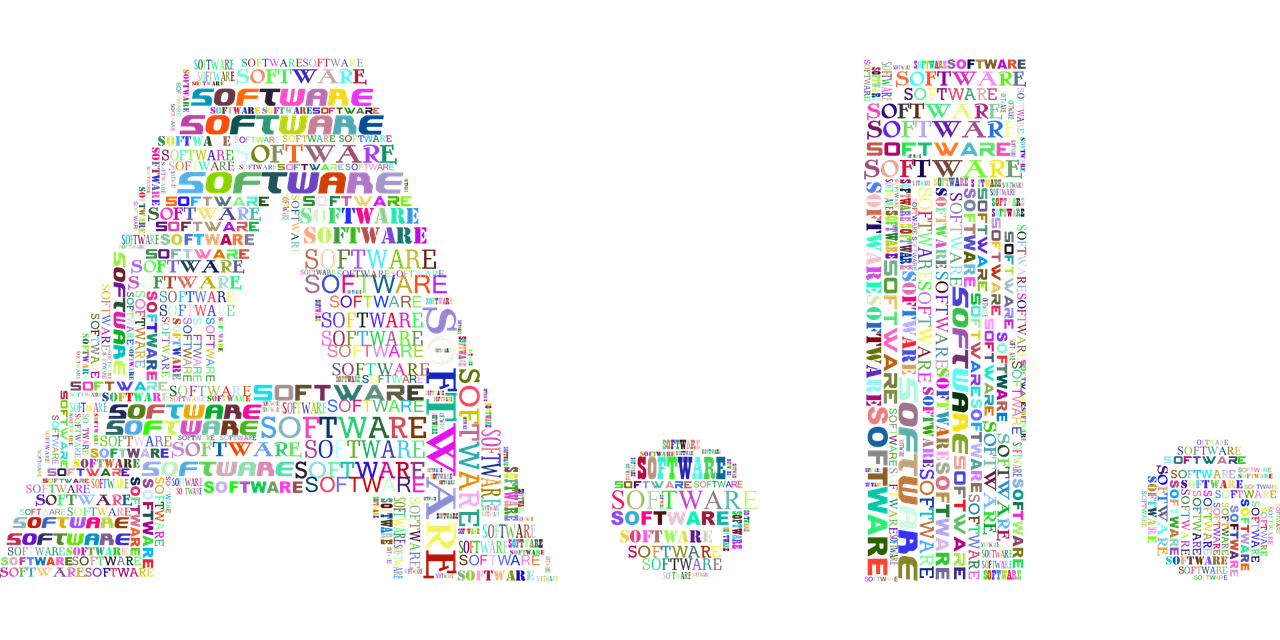